Janine Jackson interviewed Northwestern University’s Hatim Rahman about algorithms and labor for the July 5, 2024, episode of CounterSpin. This is a lightly edited transcript.
Janine Jackson: Many of us have been bewildered and bemused by the experience of walking out of a doctor’s appointment, or a restaurant, and within minutes getting a request to give our experience a five-star rating. What does that mean—for me, for the establishment, for individual workers? Data collection in general is a concept we can all grasp, but what is going on at the unseen backend of these algorithms that we should know about to make individual and societal decisions?
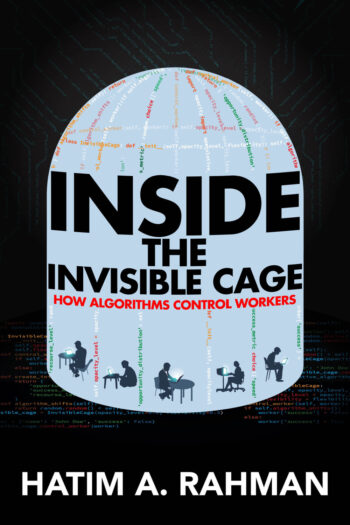
University of California Press (2024)
Hatim Rahman is assistant professor of management and organizations at the Kellogg School of Management at Northwestern University. He’s author of the book Inside the Invisible Cage: How Algorithms Control Workers, forthcoming in August from University of California Press. He joins us now by phone. Welcome to CounterSpin, Hatim Rahman.
Hatim Rahman: Thank you. I’m excited to be here.
JJ: The book has broad implications, but a specific focus. Can you just start us off explaining why you focused your inquiry around what you call “TalentFinder”? What is that, and what’s emblematic or instructive around that example?
HR: Sure, and I want to take you back about a decade ago, when I was a graduate student at Stanford University, in the engineering school, in a department called Management Science and Engineering. And at that time, when I was beginning my studies, there was a lot of talk about the future of work, and how technology, specifically algorithms and artificial intelligence, are going to lead us to the promised land. We are going to be able to choose when to work, how often we want to work, because, essentially, algorithms will allow us to pick the best opportunities and give us fair pay. And from an engineering perspective, there was this idea that it was technically feasible.
But as I began my studies, I realized that the technical features of algorithms or artificial intelligence don’t really tell us the whole story, or really the main story. Instead, these technologies really reflect the priorities of different institutions, organizations and individuals.
And so that’s kind of the through line of the book, but it was playing out in what a lot of people call the “gig economy.” Many of us are familiar with how Uber, Airbnb, even Amazon to a large extent, really accelerated this concept and the idea of the gig economy. And so you mentioned, I found this platform, which I use a pseudonym called TalentFinder, that was trying to use algorithms to create an Amazon for labor. What I mean by that is, just as you pick a product, or maybe a movie or TV show on Netflix, the thought was, if you’re looking to hire somebody to help you create a program, write a blog post, any task that you can think about that’s usually associated with knowledge work, that you could go onto this platform and find that person, again, as I alluded to earlier, just as you find a product.
And the way they were then able to do that, allow anybody to sign up to work or to find somebody, was with the use of these algorithms. And what I found, though, the reality of the situation was, that as the platform scaled, it started to prioritize its own goals, which were often in conflict, or were not shared, with workers on these platforms.
JJ: So let’s talk about that. What do you mean by that, in terms of the different goals of employers and potential workers?
HR: Sure. So it kind of went to the example you started with, that one of the thoughts was—actually, I’m going to take you back even further, to eBay. When eBay started, we take it for granted now, but the thought was, how can I trust that this person I don’t know, I don’t even know them. How can I trust that the images that they’re showing, the description that they put on, is true?
JJ: Right, right.

(via Reddit)
HR: And so eBay pioneered, really, or at least they’re the most famous example of the early company that started, like, “Hey, one way we can do this is through a rating system.” So I may take a chance and buy a product with somebody I don’t know, and if they send me what they said, I’m going to give them a five-star rating, and if they don’t, I’ll give them a lower rating.
And so since then—that was in the mid-’90s—almost all online platforms and, as you mentioned, organizations and—sorry, it is a small tangent: I was recently traveling, and I saw an airport asking me for my ratings for my bathroom experience.
JJ: Of course, yes. Smiley face, not smiley face.
HR: Exactly, exactly. Everyone copy and pastes that model. And that is helpful in many situations, but it doesn’t capture, a lot of times, the reality of people’s experiences, especially when you think about the context that I talked about. If you hired me to create a software program, and we work together for six months, there are going to be ups and downs. There are going to be things that go well, things that don’t necessarily go well, and what does that mean if you gave me a 4.8 or 4.5, right?
And so this was something that workers picked up on really early on in the platform, that these ratings, they don’t really tell the whole experience, but the algorithms will use those ratings to suggest, and people will use the search results that the algorithms curate, to make decisions about who to hire, and so on and so forth.
The problem that I traced, over the evolution of the platform, is that once workers realized that it was really important, they found out ways to game the system, essentially, to get a five-star rating all the time. And from speaking to workers, they felt this was justified, because a lot of times in an organization that hires them, they mismanage the project….
And so, in response, what the platform did, and now again almost all platforms do this, they made their algorithm opaque to workers. So workers no longer understood, or had very little understanding, of what actions were being evaluated, how they were being evaluated, and then what was the algorithm doing with it.
So, for example, if I responded to somebody faster than the other person, would the algorithm interpret that as me being a good worker or not? All of that, without notice or recourse, became opaque to them.
I liken it to, if you received a grade in class, but you don’t know why you got that grade. And, actually, many of us may have experienced this going through school; you hear this “participation grade,” and it’s like, “Wait, I didn’t know that was a grade, or why the professor gave me this grade.”
So that does happen in human life as well. One of the points I make in the book is that as we turn towards algorithms and artificial intelligence, the speed and scale at which this can happen is somewhat unprecedented.

Jacobin (2/20/18)
JJ: Right, and I’m hearing Taylorism here, and just measuring people. And I know that the book is basically engaged with higher-wage workers, and it’s not so much about warehouse workers who are being timed, and they don’t get a bathroom break. But it’s still relevant to that. It’s still part of this same conversation that’s categorically different; algorithm-driven or determined work changes, doesn’t it, the basic relationship between employers and employees? There’s something important that is shifting here.
HR: That’s correct. And you are right that one of the points that I make in the book, and there’s been a lot of great research and exposés about the workers that you mentioned, in Amazon factories and other contexts as well, that we’ve seen a continuation of Taylorism. And for those who are less familiar, that essentially means that you can very closely monitor and measure workers.
And they know that, too. They know what you’re monitoring, and they know what you’re measuring. And so they will often, to the detriment of their physical health and well-being, try to conform to those standards.
And one of the points I make in the book is that when the standards are clear, or what you expect them to do is comparatively straightforward—you know, make sure you pack this many boxes—we will likely see this enhanced Taylorism. The issue that I’m getting at in my book is that, as you mentioned, we’re seeing similar types of dynamics being employed, even when the criteria by which to grade people or evaluate people is less clear.
So, again, for a lot of people who are engaged in knowledge work, you may know what you want, but how you get there…. If you were to write a paper or even compose a speech, you may know what you want, but how you’re going to get there—are you going to take a walk to think about what you’re going to say, are you going to read something unrelated? It’s less clear to an algorithm whether that should be rewarded or not. But there is this attempt to try to, especially in trying to differentiate workers in the context that I mentioned.
So the problem with everyone having a five-star rating on eBay or Amazon, or on TalentFinder that I studied, is that for people who are trying to then use those ratings, including algorithms, it doesn’t give any signal if everyone has the same five-star rating. In situations and contexts where you want differentiation, so you want to know who’s the best comparatively to other people on the platform, or what’s the best movie in this action category or in the comedy category compared to others, then you’re going to try to create some sort of ranking hierarchy. And that’s where I highlight that we’re more likely to see what I call this “invisible cage” metaphor, where the criteria and how you’re evaluated becomes opaque and changing.
JJ: I think it’s so important to highlight the differentiation between workers and consumers. There’s this notion, or this framework, that the folks who are working, who are on the clock and being measured in this way, somehow they’re posed or pitted against consumers. The idea is that you’re not serving consumers properly. And it’s so weird to me, because consumers are workers, workers are consumers. There’s something very artificial about the whole framework for me.
HR: This is returning to one of the earlier points that I mentioned, is that we have to examine what in my discipline we call the “employment relationship.” How are people tied together, or not tied together? So in the case that you mentioned, many times consumers are kept distant from workers; they aren’t necessarily even aware, or if they are aware, they aren’t given much opportunity.
So generally speaking, for a long time, like Uber and Lyft—especially in the earlier versions of the platform; they change very rapidly—they don’t necessarily want you to call the same driver every time, [even] if you have a good relationship with them. So that’s what you mentioned, that the design of these systems sometimes keeps people in opposition with each other, which is problematic, because that’s not the technology doing that, right? That’s the organization, and sometimes the laws that are involved, that don’t allow for consumers and workers, or people more broadly, to be able to talk to each other in meaningful ways.
And in my case, on TalentFinder as well, I spoke to clients, consumers or people who are hiring these workers, and a lot of them were just unaware. They’re like, “Oh my gosh.” I highlighted in the book that they designed the rating system to say, “Just give us your feedback. This is private. We just want it to improve how the platform operates.” What they don’t tell them is that if they were to give them something slightly less than ideal, it could really imperil the workers‘ opportunity to get a next job.
We sometimes refer to this as an information asymmetry, where the platform, or the organizations, they have more information, and are able to use it in ways that are advantageous to them, but are less advantageous to the workers and consumers that are using these services.
JJ: And part of what you talk about in the book is just that opacity, that organizations are collecting information, perhaps nominally in service of consumers and the “consumer experience,” but it’s opaque. It’s not information that folks could get access to, and that’s part of the problem.

Hatim Rahman: “If you are a worker, or if you are the one who is being evaluated, it’s not only you don’t know the criteria, but it could be changing.”
HR: That’s right. It goes to this point that these technologies, they can be transparent, they can be made accountable, if organizations, or in combination with lawmakers mandating, take those steps to do so. And we saw this early on on the platform that I study, and also on YouTube and many other platforms, where they were very transparent about, “Hey, the number of likes that you get or the number of five ratings you get, we’re going to use that to determine where you show up in the search results, whether we’re going to suggest you to a consumer or a client.”
However, we’ve increasingly seen, with the different interests that are involved, that platforms no longer reveal that information, so that if you are a worker, or if you are the one who is being evaluated, it’s not only you don’t know the criteria, but it could be changing. So today, it could be how fast you respond to somebody’s message. Tomorrow, it might be how many times did you log into the platform.
And that’s problematic, because if you think about learning, the ability to learn, it fundamentally relies on being able to establish a relationship between what you observe, or what you do, and the outcome that leads to. And when that becomes opaque, and it’s so easy to change dynamically—sometimes even, let’s put aside day-to-day, maybe hour-to-hour, minute-to-minute—those really kind of supercharge the capabilities to what I call enable this dynamic opacity.
JJ: And not for nothing, but it’s clear that in terms of worker solidarity, in terms of workers sharing communication with each other, put it simple, workers need to communicate with other workers about what they’re getting paid, about their experience on the job. This is anti all of that.
HR: In related research, for my own and others, we have tried to examine this as well, especially gig work; the setup of this work makes it very difficult for workers to organize together in ways that are sustainable. Not only that, many workers may be drifting in and out of these platforms, which again makes it harder, because they’re not employees, they’re not full-time employees. And I talk to people in the book, I mentioned people, they’re between jobs, so they just want to kind of work on it.
So in almost every way, from the design of the platform to employment relationship, the barriers to create meaningful, sustainable alternatives, or resistance or solidarity, becomes that much more difficult. That doesn’t mean workers aren’t trying; they are, and there are organizations out there, one called Fairwork and others, that are trying to create more sustainable partnerships, that will allow workers to collectively share their voices, so that hopefully there are mutually beneficial outcomes.
I talked about this earlier; I mean, just to connect again with history, I think we can all agree that it’s good that children are not allowed to work in factories. There was a time when that was allowed, right? But we saw the effects that could have on the injuries, and just overall in terms of people’s development. And so we need to have this push and pull to create more mutually beneficial outcomes, which currently isn’t occurring to the same extent on a lot of these gigs and digital platforms.
JJ: Finally, first of all, you’re highlighting this need for interclass solidarity, because this is lawyers, doctors—everybody’s in on this. Everybody has a problem with this, and that’s important. But also, so many tech changes, people feel like they’re just things that happen to them. In the same way that climate change, it’s just a thing that’s happening to me. And we are encouraged into this kind of passivity, unfortunately. But there are ways to move forward. There are ways to talk about this. And I just wonder, what do you think is the political piece of this, or where are meaningful points of intervention?

Consumer Reports (5/07)
HR: That’s a great question. I do like to think about this through the different lenses that you mentioned. What can I do as an individual? What can I do in my organization? And what can we do at the political level? And, briefly, on the individual consumer level, we do have power, and we do have a voice, going back to the past, right? Consumer Reports. Think about that. Who was that started by? And that had a very influential difference on the way different industries ran.
And we’ve seen that, also, for sustainability. There’s a lot of third-party rating systems started by consumers that have pushed organizations towards better practices.
So I know that may sound difficult as well, but as I mentioned, there’s this organization called Fairwork that is trying to do this in the digital labor context.
So I would say that you don’t have to do it on your own. There are existing platforms and movements, as individuals, that you can try to tap onto, and to share these what we call again third-party alternative rating systems, that we can collectively say, “Hey, let’s use our economic power, our political power, to transact on platforms that have more transparency or more accountability, that are more sustainable, that treat workers better.” So that’s one, on the political level.
Maybe my disposition is a little bit more optimistic, but I think that we’ve seen, in the last few years, with the outsized impact social media has suggested it’s had on our discourse and politics, that politicians are more willing than before, and I know sometimes the bar is really low, but still, again, on the optimistic side, that they’re at least willing to listen, and hopefully work with these platforms, or the workers on the platforms, because, again, I really fundamentally feel that ensuring that these technologies and these platforms reflect our mutual priorities is going to be better for these organizations and society and workers in the long term as well.
We don’t want to just kick the can down the road, because of what you talked about earlier, as it relates to climate change and CO2 emissions; we’ve been kicking it down the road, and we are collectively seeing the trauma as it relates to heat exhaustion, hurricanes….
And so, of course, that should be warning signs for us, that trying to work together now, at all of those different levels, is necessary. There’s not a silver bullet. We need all hands on deck from all areas and angles to be able to push forward.
JJ: I thank you very much for that. I co-sign that 100%.
We’ve been speaking with Hatim Rahman. He’s assistant professor at Northwestern University. The book we’re talking about is Inside the Invisible Cage: How Algorithms Control Workers. It’s out next month from University of California Press. Hatim Rahman, thank you so much for joining us this week on CounterSpin.
HR: Thank you for having me.
This post was originally published on FAIR.